Business
Craig Segal Explores AI Governance: Pointers in an Evolving World
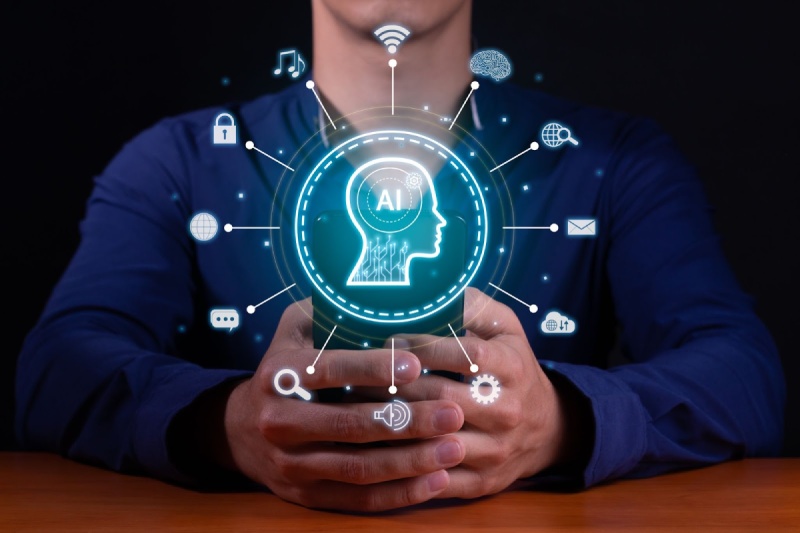
Artificial Intelligence (AI) is rapidly transforming every sector, offering opportunities for innovation, efficiency, and competitive advantage. However, the deployment of AI technologies also brings significant ethical, legal, and operational challenges. To navigate this complex area, companies must establish robust AI governance frameworks. In this article, Craig Segal explores the importance, components, and implementation strategies for effective AI governance.
The Importance of AI Governance
AI governance refers to the policies, procedures, and standards that guide the development, deployment, and monitoring of AI systems. It ensures that AI technologies are used responsibly, ethically, and in compliance with evolving regulations. The importance of AI governance can be understood through several key areas:
1. Ethical Considerations: AI systems can perpetuate biases, infringe copyright, and lead to unintended consequences. Governance frameworks help ensure that AI applications align with principles such as fairness, accountability, and transparency.
2. Regulatory Compliance: Governments and regulatory bodies are increasingly scrutinizing AI technologies. Robust governance helps organizations comply with existing laws and evolving regulations, thereby potentially avoiding legal liabilities and reputational damage.
3. Risk Management: AI systems can fail or be misused, leading to significant operational and financial risks. Governance frameworks provide mechanisms to identify, assess, and mitigate such risks.
4. Trust and Transparency: Establishing clear AI governance practices enhances stakeholder trust. Transparency in how AI systems are developed and used builds confidence among customers, employees, and partners.
5. Social Responsibility: Companies have a broad responsibility to ensure their AI applications do not harm society. Governance ensures that AI innovations contribute positively to social good.
Core Components of AI Governance
An effective AI governance framework comprises several components.
1. AI Strategy and Policy:
Vision and Objectives: Define the strategic vision for AI within the company, outlining how AI will support and enhance business goals. This vision should be communicated from the top levels of leadership down to operational teams to ensure alignment and commitment.
Policies and Guidelines: Develop comprehensive policies that cover data usage, algorithm development, deployment practices, and ethical considerations. These guidelines should be living documents, regularly reviewed and updated to reflect new insights, regulatory changes, and technological advancements.
2. Ethical Principles and Standards:
Fairness and Non-Discrimination: Ensure AI systems do not perpetuate or exacerbate biases. Implement procedures to regularly audit and mitigate bias in AI models. This includes diverse and inclusive data collection practices and the use of bias detection tools.
Accountability and Responsibility: Clearly delineate accountability for AI outcomes. Establish roles and responsibilities for AI governance across the organization, ensuring that there are designated individuals or teams responsible for monitoring and compliance.
3. Data Governance:
Data Quality and Integrity: Ensure high standards for data quality and integrity. Implement processes for data validation, cleansing, and enrichment. Data quality directly impacts the performance and fairness of AI systems.
Privacy and Security: Establish robust data privacy and security measures. Ensure compliance with data protection regulations such as GDPR and CCPA. Regular audits and security protocols should be in place to protect sensitive information and prevent breaches.
4. Risk Management:
Risk Assessment Framework: Develop a framework for assessing AI-related risks, including operational, reputational, and compliance risks. This framework should be comprehensive, covering risks across the AI lifecycle from development to deployment and beyond.
Mitigation Strategies: Implement strategies to mitigate identified risks, such as redundant systems, human-in-the-loop controls, and regular audits. Continuous risk monitoring and adaptive risk management processes are critical to addressing emerging threats.
5. Transparency:
Documentation: Maintain detailed documentation of AI models, including their design, data sources, and decision-making processes. This documentation should be accessible to relevant stakeholders and provide clear explanations of how AI decisions are made.
6. Monitoring and Continuous Improvement:
Performance Metrics: Define and track key performance indicators (KPIs) for AI systems. Regularly review their performance against these metrics to ensure they meet business objectives and ethical standards.
Feedback Loops: Establish mechanisms for continuous feedback and improvement. Incorporate lessons learned into future AI developments. This iterative approach helps refine AI systems and governance practices over time.
Implementing AI Governance
Implementing an AI governance framework requires a structured approach, involving various stakeholders and integrating governance practices into existing organizational processes. Here are key steps to effectively implement AI governance:
1. Establish Governance Structures:
AI Governance Committee: Form a cross-functional committee responsible for overseeing AI governance. Include representatives from IT, legal, privacy, compliance, and business units. This committee should meet regularly to review AI projects/direction and ensure they adhere to governance standards. They should also report AI governance practices to the executive committee and board.
Roles and Responsibilities: Clearly define roles and responsibilities for AI governance across the organization, from executive leadership to operational teams. Ensure that there are dedicated resources for governance activities, including risk management and compliance monitoring. Also, ensure that there is a decision-making framework in place.
2. Develop and Communicate Policies:
Policy Development: Develop detailed AI policies and guidelines. Ensure they cover all aspects of AI deployment, including ethical considerations, data usage, and risk management. These policies should be aligned with the organization’s broader strategic objectives and ethical standards.
Communication and Training: Communicate policies to all relevant stakeholders. Provide training to ensure understanding and adherence to governance practices. Regular training sessions and workshops can help embed governance principles into the organizational culture.
3. Integrate Governance into AI Lifecycle:
Design and Development: Incorporate governance principles into the AI development process. Use ethical design frameworks and conduct thorough testing and validation. Engage stakeholders throughout the development process to ensure alignment with governance standards.
Deployment and Monitoring: Implement robust monitoring mechanisms to ensure compliance with governance standards throughout the AI system’s lifecycle. Continuous monitoring and post-deployment evaluations are crucial for maintaining governance.
4. Leverage Technology and Tools:
Governance Platforms: Utilize AI governance platforms and tools that provide capabilities for model monitoring, risk assessment, and compliance tracking. These platforms can automate many governance tasks, improving efficiency and accuracy.
Automation and Analytics: Leverage automation and analytics to streamline governance processes, such as continuous monitoring and anomaly detection. Advanced analytics can provide deeper insights into AI system performance and identify potential governance issues.
5. Engage with External Stakeholders:
Regulatory Bodies: Maintain open communication with regulatory bodies. Participate in industry forums and contribute to the development of AI governance standards. Staying engaged with regulators helps anticipate regulatory changes and align internal practices accordingly.
External Audits: Engage third-party auditors to review and validate AI governance practices. Use their insights to strengthen governance frameworks. External audits provide an unbiased assessment of governance effectiveness and identify areas for improvement.
Challenges and Future Directions
Implementing AI governance is not without challenges. Organizations may face issues such as the complexity of AI systems, evolving regulatory landscapes, and the need for specialized expertise. To address these challenges, companies should:
1. Stay Informed: Keep abreast of developments in AI technology and regulations. Regularly update governance practices to reflect the latest trends and requirements. This proactive approach ensures that governance frameworks remain relevant and effective.
2. Invest in Skills: Develop internal expertise in AI governance. Invest in training and development programs to build a skilled workforce capable of managing AI risks and compliance. Consider hiring or training professionals with backgrounds in data science, ethics, and legal compliance.
3. Innovate Responsibly: Balance innovation with responsibility. Encourage a culture of ethical AI development and use within the organization. Responsible innovation ensures that AI advancements are aligned with societal values and contribute positively to the community.
4. Adaptability and Scalability: Design governance frameworks that are adaptable and scalable. As AI technologies evolve, governance practices should be flexible enough to accommodate new developments and scalable to handle increasing complexity and deployment scale.
5. Stakeholder Engagement: Foster engagement with a broad range of stakeholders, including employees, customers, partners, and the broader community. Incorporate their perspectives and concerns into governance practices to ensure comprehensive and inclusive governance.
6. Ethical Review Boards: Where applicable, establish ethical review boards to oversee the deployment of AI systems. These boards can provide independent oversight and ensure that AI applications align with ethical standards and societal expectations.
7. Transparency Initiatives: Enhance transparency through initiatives such as publishing AI ethics reports and creating open channels for feedback. Transparency initiatives build trust and demonstrate the organization’s commitment to ethical AI use.
In conclusion, AI governance is crucial for ensuring that AI technologies are used responsibly and effectively within organizations, and ultimately reducing associated risks. By establishing governance frameworks, companies can mitigate risks, comply with regulations, and build trust with stakeholders. As AI continues to evolve, so too must governance practices, ensuring that organizations are well-positioned to harness the full potential of AI while safeguarding ethical and societal values. The journey towards effective AI governance is ongoing, requiring continuous learning, adaptation, and commitment to evolving principles.
Dive deeper into the world of tech law. Head over to the Craig Segal Toronto Tech Law blog for insightful articles.
-
Entertainment2 weeks ago
Album “Short n’ Sweet” by Sabrina Carpenter Hits at No. 1 on the Billboard 200, Surpassing Travis Scott
-
Health3 weeks ago
Dr. Tony Nader Reveals the Fundamental truth : ‘Conciousness Is All There Is’ Uncovers the Cosmic Association Between Modern Science and Vedic Knowledge
-
Business3 weeks ago
General Motors’ Cruise and Uber Technologies Agree to a Multi-year Partnership to Offer Driverless Rides Next Year
-
Health4 weeks ago
The Art of Healing: Dr. Meleeka Clary’s Impact on Mental Health and Human Rights
-
Tech2 weeks ago
Top 10 Marketing Services for Plumbers
-
Business4 weeks ago
14 Tips to Grow Your Business Using E-Commerce Email Marketing
-
World3 weeks ago
Second Largest Diamond in the World is Discovered in Botswana
-
Tech4 weeks ago
2025 Volkswagen ID.Buzz will Become VW’s Most Expensive Model in the United States, Starting at $61,545 and Possibly Exceeding $71K